5 Pitfalls in data adoption in PE and how to overcome them
Tobin Sydneysmith & Hugo Pinto | 11th July 2023
The Private Equity (PE) industry is at a fascinating stage of maturity in its adoption of data analysis and AI journey, with numerous potential challenges and a significant opportunity to gain advantages over competitors; the industry is in a period of rapid change.
Amidst this transformative landscape, PE firms strive to progressively orient their strategies towards harnessing more complete data and leveraging predicting analytics to enrich their investment decisions, drive operational efficiencies and unlock value within their portfolio companies.
While the ability to effectively leverage larger datasets has become an indispensable tool for PE firms seeking to gain an edge and prosper in the contemporary marketplace, there are several challenges or pitfalls in data adoption for these firms.
Here are some of them and ways to overcome them:
Data-Driven origination
On the one hand, there is growing pressure from Limited Partners (LPs) to have more continuous and detailed insights on how their investments are performing and how the decision to deploy capital is made – over 2/3s of them now expect a data-driven origination strategy to be in place. On the other hand, the influx of data talent into PEs has doubled in the last two years, and 90% of PE professionals who responded to a survey by S&P Global said that they agree that increased competition for deals in leading to a tighten focused on innovating their technology and data approaches.
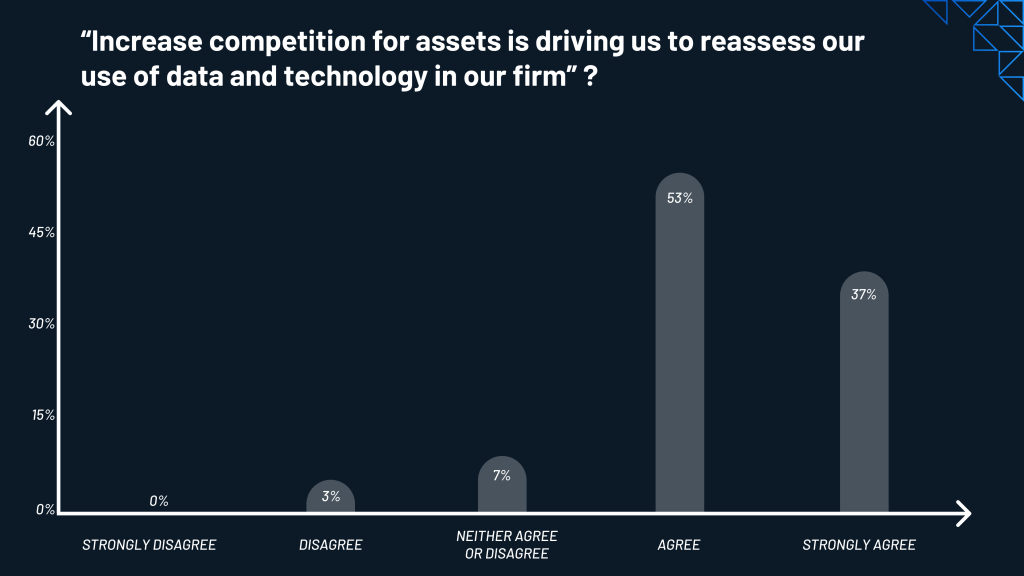
Source: S&P Global Market Intelligence
The time is now
While speaking to our clients, we often hear they need to arrive at sourcing conversations and origination meetings earlier, and that timing is key to winning business. They also tell us that having the proper insight about the company, and the right context to offer the right inputs that can help build value in the relationship, is invaluable and differentiating. This automatically opens up a few challenges for both investment and origination teams:
-
- How can they sift through the ever-changing landscape of investable targets to spend their energies on the right prospects?
-
- What’s the best way to contact them, and are there any personal connections within your firm?
-
- How do you know the right moment to contact each individual company when you are keeping track of a universe of a million companies in your potential target list?
Especially in a challenging market, the value of having the correct information is game-changing, and it can make the difference in increasing efficiency in managing vast pipelines or companies, especially in trendy sectors such as ESG, Deeptech and Medtech, where things change at a tremendous pace.
The key challenges
To succeed at adopting in-house data processing, extracting valuable insights and applying effective Machine Learning practices – there are a few key challenges we typically help our clients address:
1. There’s a plethora of SaaS tools and data vendors, but an investor can only build a competitive advantage by bringing data processing in-house.
2. Buy or build? Building an in-house capability requires considerable commitment. How does an in-house experiment get operationalised?
3. Many SaaS vendors are providing insights and even integrating into Dealflow, but the PE firm retains no IP
4. Multiple data vendors with Varying accuracy, coverage and depth
5. Difficulty in personalising the corpus of data to match your business objectives and with your parameters to identify suitable business opportunities, differently from everyone else that is also using the same data & tools
6. Getting users to adopt them and change their ways of working
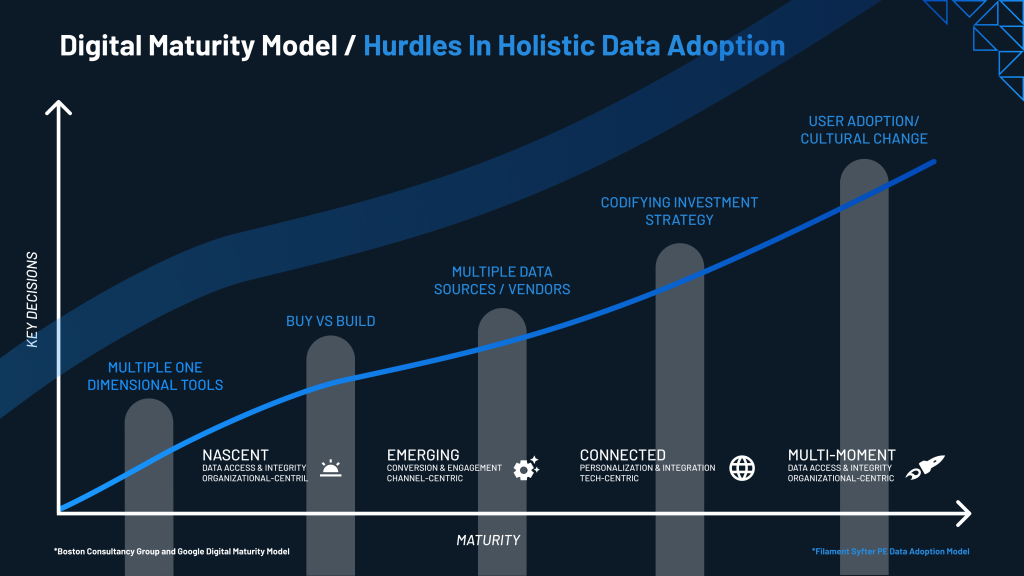
The latter is clearly the most significant challenge; that’s where having a Project or product management team focused on tech deployment and adoption makes all the difference.
How these can be avoided
Ensuring you have the right combination of data vendors and the right balance on internal and external data sources is one of the keys to having the right coverage across regions and sectors and against the parameters of the niches you’re looking for.
However, even after collecting and cleaning the data, effective prioritization and matching are paramount to surface the correct information at the right time. Syfter matches against third-party data sources, structure and unstructured data, merging conventional vendors with alternative data. Some sources will be clean, others messy, but we employ our cutting-edge technology to find the relevant assets and groups in the support structure our clients want to introduce.
Moreover, integration with CRM and top-of-pipe market intelligence ensures everyone has a single source of truth, and all sourcing/origination work is done with the richest information available.
To address the cultural and behavioural change required to make any of this a success, having a strong internal sponsor, garnering the needs of key stakeholders involved from the beginning is essential. Spending time understanding how they make decisions, use the tools, and use the information daily, and only then solving how they can do that more efficiently.
Underestimating the complexity of data transformation:
Organizations need to recognize the complexity of implementing a data-driven approach, as underestimating the time, cost, and resources required can lead to unfinished projects or not meeting expected returns on investment. To avoid this, proper planning and resource allocation is essential for data-related initiatives. A phased approach may also be beneficial rather than a significant transformation simultaneously.
It’s a complex exercise to balance the technical, functional and behavioural needs to make this a success. Still, there is clearly momentum in adopting more data in decision-making across Private equity and overall private markets.
One of our clients has managed to identify three companies and deploy capital worth 100x for their investment in data.
We would love to help you achieve the same outcome.
Get in touch!
Tobin Sydneysmith
tobin.sydneysmith@filament.ai
Head of Product Marketing