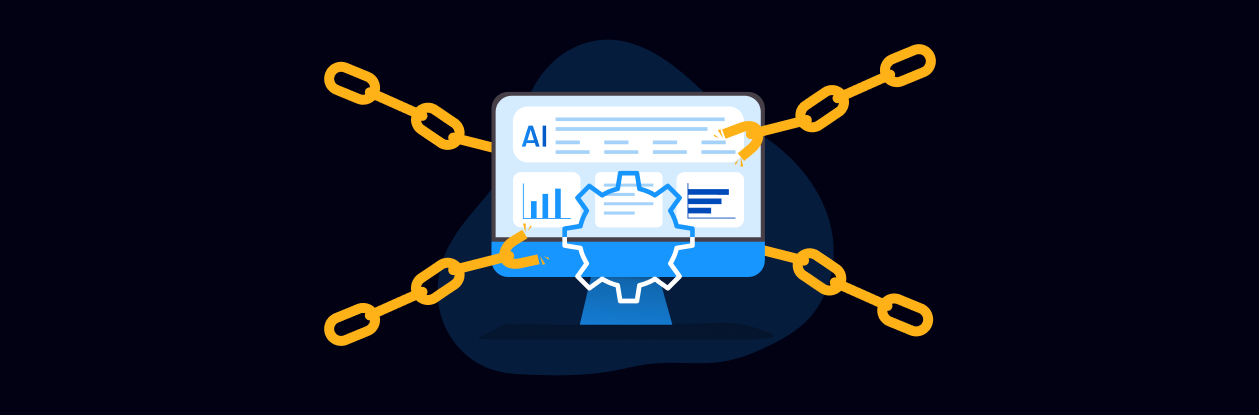
Looking Beyond The Hype: Unlocking AI’s Potential in Private Equity in 2024
Introduction: GenAI’s Awkward Teenage Years
Since the launch of ChatGPT in December 2022, the world has been buzzing about AI. From promises of fully self-driving cars to intelligent drug discovery, the potential applications seem limitless. The PE sector is no exception with firms eagerly embracing AI to help them manage their portfolio.
However, the Gartner AI Hype Cycle for June 2024 now places Generative AI, the tech that started the current frenzy, as sliding down into the “Trough of Disillusionment.” Investments into early-stage AI companies are drying up as initial excitement fades and many AI Proofs of Concept (POCs) fail to deliver an ROI or land companies in hot water. A recent market crash is widely believed to have been caused by poor results from tech firms who have invested heavily in AI and are yet to see returns. The reality is that deploying and scaling AI solutions beyond POCs is complex. It requires specialized expertise in machine learning and data science, not just a 5 minute conversation with a chatbot.
However, there’s a huge silver lining to these recent events. As the hype cycle settles, we’re entering a period where focus can now shift towards practical, real-world applications and sustainable value creation. And, we can do all of that without worrying about potential disruption from the next GPT model in the short term. In this new era, PE firms that see through from the hype and embrace AI strategically and methodologically will gain a significant competitive advantage. Let’s explore how AI can be leveraged to unlock new levels of efficiency, insight, and success in 2024.
Foundation Models: The Starting Point for PE’s AI Journey
The allure of readily available AI tools and APIs is undeniable. Foundation models like GPT-4, Google Gemini and Anthropic Claude offer a quick and easy way to integrate AI capabilities, allowing PE firms to quickly build POC projects leveraging LLM technologies. Hallucination, the tendency for models to confidently bluff answers to questions if they don’t know the answer, remains an issue but can be minimized through techniques like Retrieval Augmented Generation. The big cloud providers already have off-the-shelf templates for RAG: AWS Kendra, Azure AI Search and Google Cloud AI Search.
Whilst it’s easy to get started with these tools and models, as previously discussed, POC AI systems are very difficult to scale out in production without some help from experienced data science and data engineering teams. Many PE firms will be experimenting with these tools at this level. To truly stand out and get a competitive advantage, firms need to go further than building PoCs on top of foundational models and build upon them with their own proprietary datasets, expertise and investment strategies.
Building In-House Expertise or Partnering for Success
As the PE sector continues to navigate the complex landscape of AI adoption, many firms are faced with a crucial decision: build an in-house AI team or partner with a reputable AI software vendor. While having dedicated experts on staff can be beneficial, it comes with significant costs and resource commitments. Moreover, the rapid evolution of AI technology means that internal teams must continually invest in training and upskilling to stay current – a challenge even for large organizations.
Fortunately, the fintech supply chain is rapidly incorporating AI capabilities, making it easier than ever for firms to leverage these benefits without needing to develop their own expertise or infrastructure. CRMs and market intelligence platforms like Syfter are now providing AI capabilities that work out of the box during setup and which can be quickly tailored to a specific firm’s investment thesis, industry focus, and growth strategy.
As the PE sector continues to navigate the complex landscape of AI adoption, partnering with reputable AI software vendors can provide a more agile and cost-effective solution, allowing firms to unlock the full potential of AI without sacrificing resource commitments. With the right partnerships and expertise in place, PE firms are now poised to unlock the full potential of AI, which begins with harnessing high-quality data.
Data: The Fuel for AI Success
Even in the post-ChatGPT world, the success of AI-powered decision-making hinges on one crucial factor: high-quality data. Just as a car requires gasoline to run, successful AI projects rely on high quality historical data to help them to understand how a firm operates, the sectors they work with and the kinds of deals they like to make. Foundation models like GPT-4 are pre-trained on huge volumes of data collected from various sources across the internet but they have limited knowledge of the private investment industry and no knowledge of individual firms’ preferences.
Building such a dataset might sound like a scary undertaking and indeed, manually building and cleaning this dataset is a lot of work, even for an experienced data engineering team. However the right tools can give firms a head start.
A lot of firms’ most valuable data usually sits in their CRM system and much of the auxiliary/complementary data like financials, market trends and industry news can be purchased and integrated with internal knowledge to provide comprehensive coverage of entire sectors and markets. Tools like Syfter can automate a lot of the hard work through integrations with a wide range of CRM systems and external data services. Firms can then take advantage of models that come integrated as part of the platform that have been fine-tuned for specific use cases in the private equity industry and which can be further trained and customized using proprietary data to give them an edge in the market.
AI Applications in Private Equity
The Private Equity sector has been slow to adopt AI, often overlooking practical use cases in favor of more glamorous chatbot and question-answering systems. However, beyond POC, the complexity of building systems that attempt to handle open-ended questions without hallucination often leaves users disappointed with the results. Instead, we recommend focusing on AI applications in private equity that can be quality-controlled and measured for effectiveness. This approach enables firms to harness the full potential of AI and drive business value through improved efficiency, accuracy, and decision-making.
Deal sourcing and due diligence in private equity can be enhanced with AI by leveraging predictive modeling to analyze historical data on companies similar to those previously dealt with. Additionally, large language models (LLMs) can be used to translate documents, extract information from company profiles, and explain differences between companies with similar profiles. This can help identify promising opportunities more quickly and make informed investment decisions that drive business value.
Effective portfolio management in private equity requires proactive decision-making to ensure the continued growth and success of invested companies. AI can be leveraged through predictive modeling, analyzing historical data on financial performance and market trends, and through Natural Language Processing (NLP) models that monitor industry news for positive indicators like awards or negative signs like scandals. This enables the prediction of future success or potential issues, allowing private equity firms to make informed decisions and drive business value and growth.
Syfter can be configured to provide regular email summaries of market activities, providing an additional tool for monitoring industry developments and staying ahead of the competition.
By focusing on specific use cases and quality-controlled applications, private equity firms can unlock the full potential of AI and drive business value through improved efficiency, accuracy, and decision-making.
Conclusion
As we navigate the complexities of the post-ChatGPT world, it’s clear that AI has become an integral part of the private equity landscape. While the hype cycle may be settling, the potential benefits of AI in PE are undeniable.
By focusing on practical applications and sustainable value creation, private equity firms can unlock new levels of efficiency, insight, and success in 2024 and beyond. This requires a strategic approach to AI adoption, building upon foundation models with proprietary datasets, expertise, and investment strategies.
As the fintech supply chain continues to evolve, partnering with reputable AI software vendors like Syfter can provide a cost-effective solution for firms looking to unlock the full potential of AI without sacrificing resource commitments.
Ultimately, the key to success lies in harnessing high-quality data to drive business value through improved efficiency, accuracy, and decision-making. By focusing on specific use cases and quality-controlled applications, private equity firms can make informed investment decisions, predict future success or potential issues, and drive business growth.
As we enter this new era of AI adoption, one thing is clear: the PE sector that sees through from the hype and embraces AI strategically and methodologically will gain a significant competitive advantage. The time to start unlocking the full potential of AI is now.